Pioneering the Future of Decentralized Intelligence
Imagine a network of specialized AI agents working together across a secure, decentralized architecture. Each agent handles specific tasks, communicates effortlessly, and operates autonomously—enabling your business to innovate, streamline processes, and make data-driven decisions in real-time.
“Just as DNS revolutionized the internet by providing a neutral framework for web access, we need a similar infrastructure for the “Internet of Agents.” We’re launching NANDA – an open-protocol for registry, verification, and reputation among AI agents – in collaboration with national labs and global universities (decentralized across 8 time zones!)
NANDA will pave the way for seamless collaboration across diverse systems, fully compatible with enteprise protocols like MCP and A2A. This initiative is a step toward democratizing agentic AI, creating an ecosystem where specialized agents can work together to solve complex challenges—just like DNS did for the web.”
Ayush Chopra
PhD Candidate at MIT
This dynamic ecosystem operates within a secure, decentralized infrastructure that ensures privacy, trust, and accountability at every level. This concept is brought to life through the NANDA (Networked Agents And Decentralized AI) initiative, which aims to create a truly decentralized Internet of AI Agents.
The Internet of AI Agents
At the MIT Decentralized AI Summit, the Model Context Protocol (MCP) was introduced as a standardized method for enabling communication between AI agents, tools, and resources. While MCP serves as a foundational interaction protocol, NANDA goes beyond the basics by addressing the infrastructural challenges required to support a truly decentralized, large-scale network of AI agents.
NANDA builds upon MCP to provide the critical components needed for a distributed ecosystem where potentially billions of AI agents can collaborate across organizational and data boundaries. The protocol extends the capabilities of traditional AI systems, fostering seamless agent collaboration at scale—something that current centralized models struggle to achieve due to rigid data structures and lack of transparency.
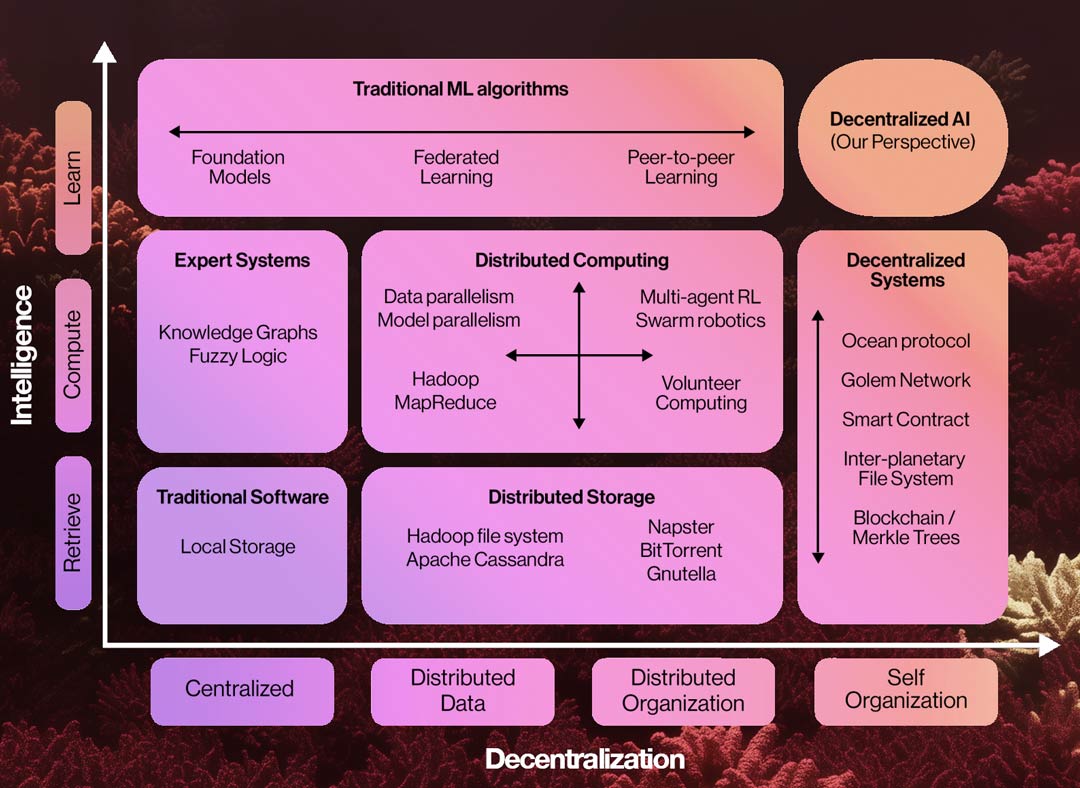
“Enter the landscape of existing paradigms and the path towards decentralized AI. ML algorithms like foundation models excel in AI capabilities but remain centralized. Decentralized systems, like blockchains and volunteer computing, distribute storage and computation but lack intelligence. We argue that bringing the two capabilities together can have an outsized impact. We call upon the AI community to focus on the open challenges in the upper-right quadrant, where decentralized architectures can give rise to anew generation of AI systems that are both highly capable and aligned with the values of a decentralized society.”
A Perspective on Decentralizing AI
Abhishek Singh, Charles Lu, Gauri Gupta, Nikhil Behari, Ayush Chopra, Jonas Blanc, Tzofi Klinghoffer, Kushagra Tiwary, and Ramesh Raskar
MIT Media Lab
Everyman Metaphor
Imagine a vast coral reef ecosystem.
Each coral polyp, tiny but specialized, is like an individual AI agent in this massive decentralized network. Some filter nutrients, others build the reef, and still others host symbiotic relationships with fish, algae, and crustaceans—each with its unique role.
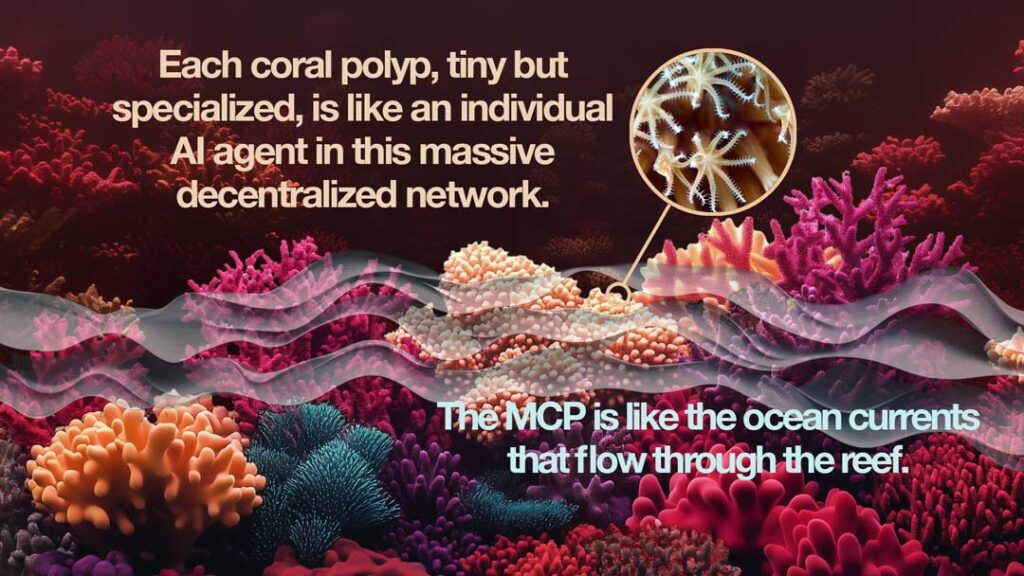
Similarly, AI agents in NANDA perform specific tasks—learning, navigating, transacting, and interacting—each contributing to the broader ecosystem.
The Model Context Protocol (MCP) is similar to the ocean currents that flow through the reef. These currents are consistent, structured, and essential—they allow nutrients, larvae, and signals to move through the system. In the same way, MCP ensures that information flows smoothly, securely and predictably between agents, tools, and resources.
But ocean currents alone don’t make a thriving reef.
That’s where NANDA comes in—it’s the reef structure itself, the intricate, interconnected framework built over time that supports the life within. NANDA provides the scaffolding—the decentralized architecture—where all these AI agents (like reef dwellers) can thrive together. It allows for scalability, resilience, and collaboration across countless agents, just like a healthy reef sustains an immense variety of life.
So in this metaphor:
- AI agents = reef creatures and coral polyps
- MCP = ocean currents and nutrient flows
- NANDA = the coral reef’s skeleton, enabling life to flourish at scale
Together, they form a self-sustaining, adaptive ecosystem—an Internet of AI agents as vibrant and alive as a coral reef teeming with collaborative intelligence.
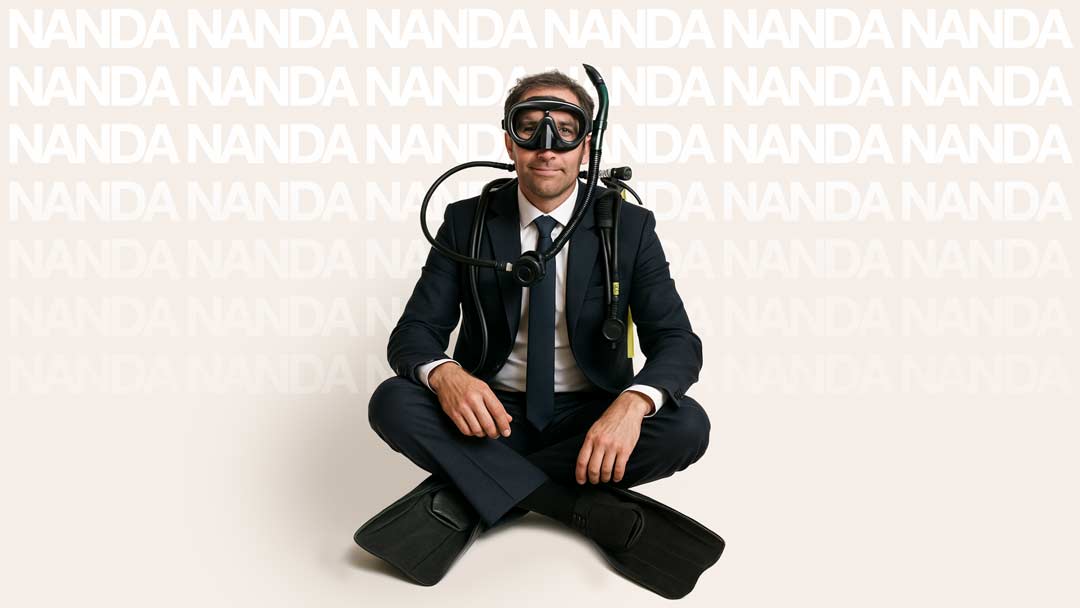
Why NANDA Matters to a CEO
Strategic Advantage
Adopting NANDA positions organizations to lead in AI-driven markets by supporting everything from R&D to regulated processes. Its infrastructure enables flexibility for creative automation and ensures reliability for mission-critical applications. By aligning AI maturity with business goals, NANDA facilitates a smoother path to AI adoption, providing long-term competitive advantages in industries where agility and scalability are key.
Decentralized Intelligence at Scale
NANDA’s approach transforms traditional AI systems by decentralizing both the data and control, enabling an intelligent ecosystem of agents that collaborate seamlessly. This enables secure, dynamic workflows across industries, from healthcare to finance. Unlike standalone AI systems, NANDA offers enhanced capabilities for discovery, search, authentication, and interaction traceability—ensuring secure and scalable intelligence for enterprise environments.
Innovation with Governance
With NANDA, organizations can embrace innovation while maintaining full control over security and compliance. NANDA balances rapid development with the need for governance by providing developers with tools for building secure, verifiable applications and agents. Secure authentication protocols and verifiable interaction logs (“Trace”) ensure that the system remains accountable, transparent, and aligned with regulatory standards for sensitive operations.
Why NANDA Will Quickly Provide a Secure Solution
For institutions like hospitals or financial organizations, adopting a decentralized system like NANDA may initially raise concerns regarding security and compliance. However, NANDA is designed to address these concerns head-on. Built from the ground up with trust and accountability at its core, NANDA leverages secure multi-layered encryption, authentication mechanisms, and immutable trace logs to ensure the integrity of data and interactions.
Additionally, NANDA’s infrastructure is designed to scale while meeting the most stringent privacy and regulatory requirements. By incorporating real-time verification, verifiable agent-to-agent interactions, and decentralized control, NANDA provides a robust security framework that enables organizations to trust its decentralized agents with mission-critical tasks while ensuring compliance with industry standards. The framework’s focus on decentralized trust eliminates the need for a single point of failure, further strengthening its suitability for high-security environments like healthcare or finance.
Core Value Proposition and Enabling Technology
NANDA explicitly positions itself as not just an interaction protocol (like MCP) but as a comprehensive infrastructure designed to support decentralized, large-scale AI collaboration. By providing a network fabric with critical components such as:
- Registries for discovering agents, tools, and resources
- Interaction databases for auditing and referencing agent interactions
- Developer tools and SDKs to integrate third-party applications
NANDA creates the foundation for building a secure, scalable ecosystem where AI agents can collaborate across industries with confidence.
Key Differentiation Factors in the AI Agent Ecosystem
NANDA distinguishes itself from other AI agent frameworks through its explicit focus on decentralization, its large-scale infrastructure, and its strong academic foundation. By prioritizing decentralized trust, NANDA addresses the core limitations of centralized AI models and networks. Furthermore, NANDA’s traceable accountability systems ensure that every action is verifiable, creating a trustworthy environment for enterprise-scale applications.
Unlike frameworks like LangChain or AutoGen, which focus on individual agents or small-team coordination, NANDA aims to build the “interstate highway system” for decentralized AI—creating the infrastructure needed for billions of agents to collaborate seamlessly across the globe. This vision, coupled with a deep academic research foundation, positions NANDA as a true leader in the development of decentralized intelligence.
Elevator Pitch (AI Strategy Lens):
NANDA is a secure AI framework that helps businesses innovate with confidence. It connects specialized AI agents across a decentralized network, enabling them to collaborate, learn, and make decisions autonomously. Built on Anthropic’s MCP, NANDA offers strong security with encrypted communication, real-time authentication, and verifiable logs, ensuring that sensitive operations stay secure and compliant. With easy integration and developer tools, NANDA supports rapid innovation, making it a scalable and reliable solution for your business to harness AI safely and effectively.
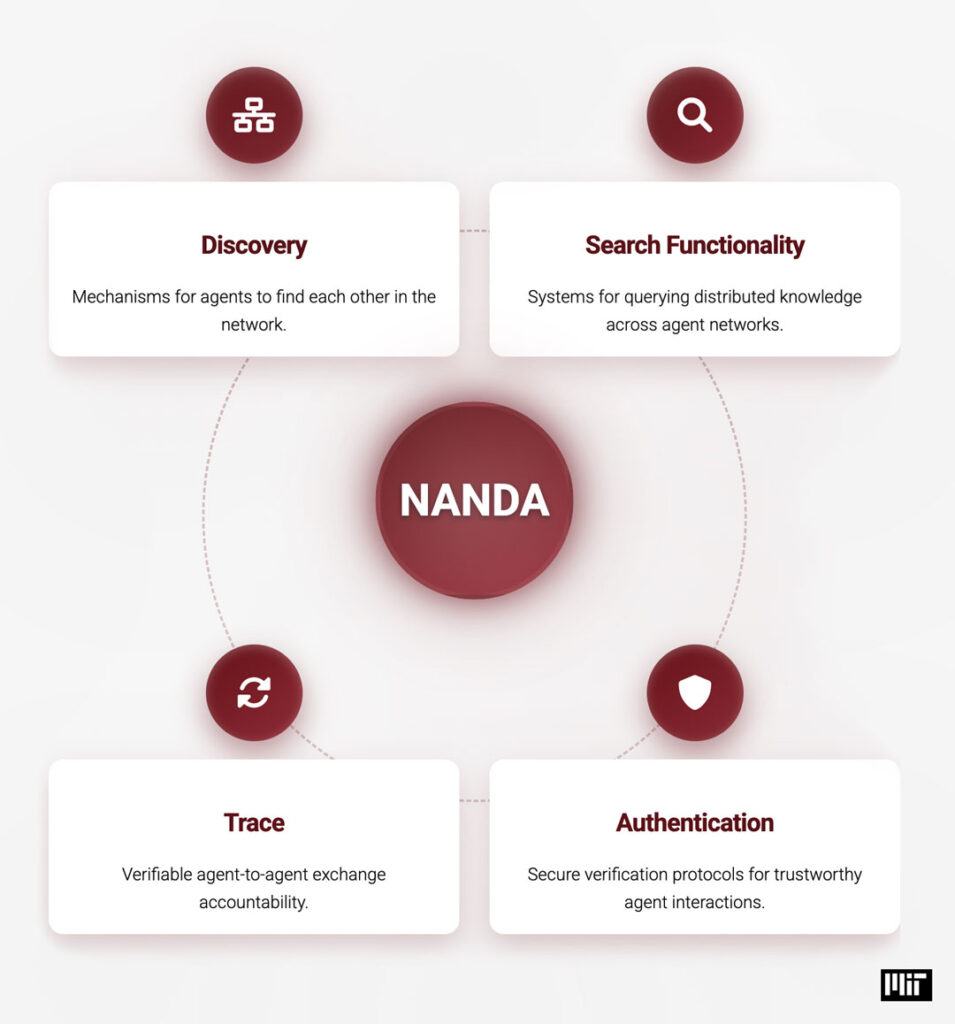
NANDA Ecosystem
Discovery within the NANDA ecosystem enables agents to find and interact with one another efficiently across the network. This includes robust Search Functionality for querying distributed knowledge, and Authentication mechanisms to ensure secure and trustworthy agent interactions. A Trace layer supports verifiable accountability in agent-to-agent exchanges. The system is built on a modular architecture comprising a Protocol Layer that forms the foundation for AI communication, Developer Tools to empower builders within the ecosystem, an Infrastructure Layer maintaining a registry of agents, resources, and interactions, and a suite of Applications that support third-party integrations via SDKs, registries, and databases.
SOURCE:
General Information