The fascinating journey of AI video generation, tracing the pioneers, exploring cutting-edge techniques, and discovering modern applications.
Introduction: The realm of artificial intelligence (AI) has witnessed remarkable advancements in recent years, with one of its most intriguing applications being AI video generation. This blog post embarks on a comprehensive journey through time, unraveling the history, techniques, pioneers, modern applications, and ethical implications of AI video generation.
The Genesis of AI Video Generation: In its nascent stages, AI video generation faced formidable challenges. Early attempts often resulted in crude outputs, plagued by artifacts and inconsistencies. However, the landscape began to shift with the advent of neural networks, particularly deep learning architectures.
Early Attempts and Limitations: Early attempts at AI video generation were constrained by computational limitations and the lack of sophisticated algorithms. Rule-based systems struggled to capture the complexity and nuances of natural video scenes, leading to unsatisfactory results characterized by artifacts and distortions.
Emergence of Neural Networks: The emergence of neural networks, fueled by advances in hardware and algorithmic innovation, heralded a new era of possibilities for AI video generation. Deep learning architectures, characterized by multi-layered networks capable of hierarchical feature representation, offered unprecedented capabilities in synthesizing realistic video content.
Pioneers in AI Video Generation: Among the trailblazers in this domain is Ian Goodfellow, whose pioneering work on Generative Adversarial Networks (GANs) revolutionized AI-generated content. GANs, through a competitive process between a generator and a discriminator, excel in synthesizing realistic images and videos.
Ian Goodfellow: GANs and Image Synthesis: Ian Goodfellow’s seminal paper on Generative Adversarial Networks (GANs) laid the foundation for a new paradigm in AI video generation. By pitting a generator network against a discriminator network in a game-theoretic framework, GANs achieve remarkable proficiency in synthesizing realistic images and videos.
Alexey Dosovitskiy: Video Prediction Networks: Another luminary in the field is Alexey Dosovitskiy, whose research on Video Prediction Networks (VPNs) paved the way for predictive modeling of dynamic scenes. By leveraging recurrent neural networks (RNNs) and convolutional layers, VPNs demonstrate remarkable proficiency in generating coherent video sequences.
Fei-Fei Li: Advancements in Computer Vision: Fei-Fei Li, renowned for her contributions to computer vision, has significantly influenced AI video generation through advancements in perceptual understanding and scene understanding. Her research endeavors have enhanced the fidelity and realism of AI-generated videos.
Techniques Behind AI Video Generation: At the heart of AI video generation lie sophisticated techniques, chief among them being Generative Adversarial Networks (GANs). GANs employ a dual-network architecture, wherein the generator fabricates data samples while the discriminator distinguishes between real and synthetic data.
Generative Adversarial Networks (GANs): Generative Adversarial Networks (GANs) form the bedrock of AI video generation, leveraging adversarial training to generate realistic video content. Through an iterative process of refinement, GANs learn to synthesize video sequences that exhibit high fidelity and perceptual realism.
Variational Autoencoders (VAEs): Variational Autoencoders (VAEs) enable the synthesis of diverse and high-fidelity video content by modeling data distribution in a latent space. By encapsulating data variability in a probabilistic framework, VAEs facilitate the generation of novel video sequences with controllable attributes.
Recurrent Neural Networks (RNNs) and Long Short-Term Memory (LSTM): Recurrent Neural Networks (RNNs) and LSTM networks excel in capturing temporal dependencies, facilitating the generation of coherent and contextually rich video sequences. Their recurrent nature enables the synthesis of video content with long-term coherence and contextual relevance.
Transformer Models: Transformer models, exemplified by architectures such as the Transformer and its variants, have emerged as powerful tools for AI video generation. By leveraging self-attention mechanisms and parallel processing, Transformer models excel in capturing long-range dependencies and modeling complex interactions within video sequences.
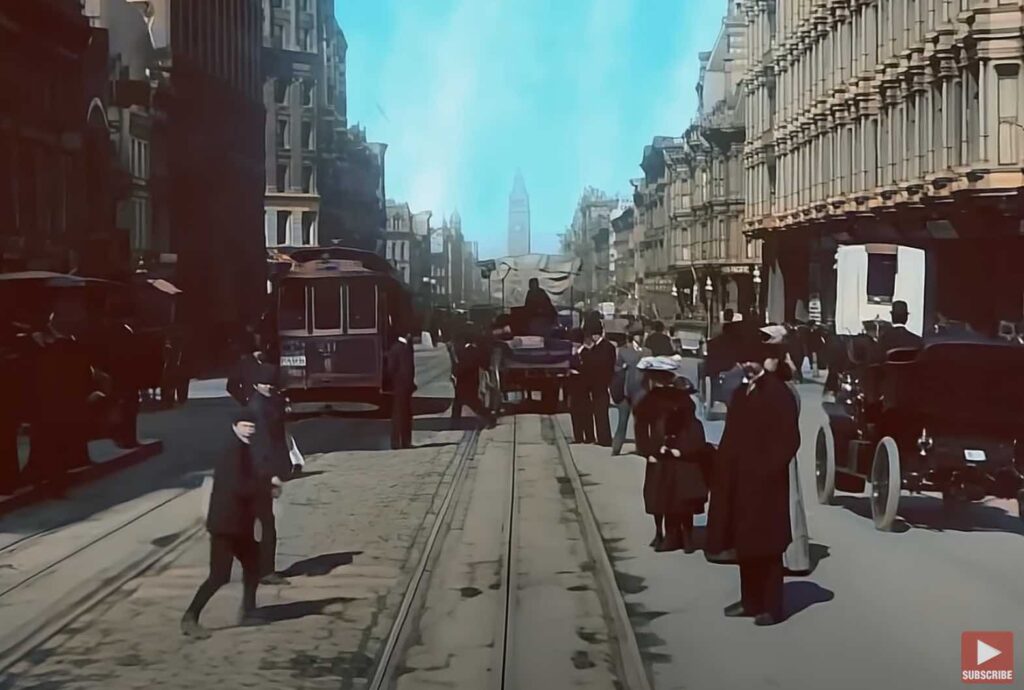
Modern Applications of AI Video Generation: The proliferation of AI video generation has engendered a plethora of modern applications, spanning diverse domains ranging from entertainment to education and beyond.
Deepfake Technology: Deepfake technology, fueled by AI algorithms, has redefined the landscape of video manipulation, blurring the lines between reality and fabrication. From creative endeavors in filmmaking to malicious manipulation in disinformation campaigns, deepfakes have elicited both awe and apprehension.
Video Enhancement and Restoration: AI-driven video enhancement and restoration techniques offer a new lease of life to archival footage, mitigating noise and enhancing visual quality for diverse applications. From digital preservation to forensic analysis, these advancements hold immense potential in enhancing the accessibility and fidelity of video content.
Virtual Reality and Augmented Reality: The fusion of AI video generation with virtual and augmented reality heralds a new era of immersive experiences, redefining the boundaries of storytelling and simulation. From lifelike simulations to interactive storytelling, AI-generated videos enrich the fabric of virtual environments, transcending conventional boundaries.
Film and Entertainment Industry: The film and entertainment industry has embraced AI video generation as a powerful tool for content creation and storytelling. From visual effects and CGI rendering to virtual actors and dynamic scene generation, AI-driven technologies are reshaping the creative landscape and pushing the boundaries of imagination.
Education and Training: In the realm of education and training, AI video generation holds immense promise for immersive learning experiences and skill development. From interactive tutorials and simulations to personalized learning environments, AI-generated videos empower learners with engaging and impactful educational content.
Ethical and Societal Implications: Amidst the proliferation of AI video generation, concerns pertaining to ethics and societal impact loom large. The rampant misuse of deepfake technology for malicious purposes underscores the urgent need for robust safeguards and regulatory frameworks.
Misuse of Deepfake Technology: The misuse of deepfake technology underscores the urgent need for robust safeguards and ethical guidelines to mitigate the proliferation of misinformation and malicious manipulation. From imperson