AI is evolving fast, and a new approach is gaining traction: agentic AI. Unlike current AI tools like ChatGPT that need human input to act, agentic AI is designed to work independently. The idea is that it could handle complex tasks—like scheduling or predicting equipment needs—without waiting for instructions.
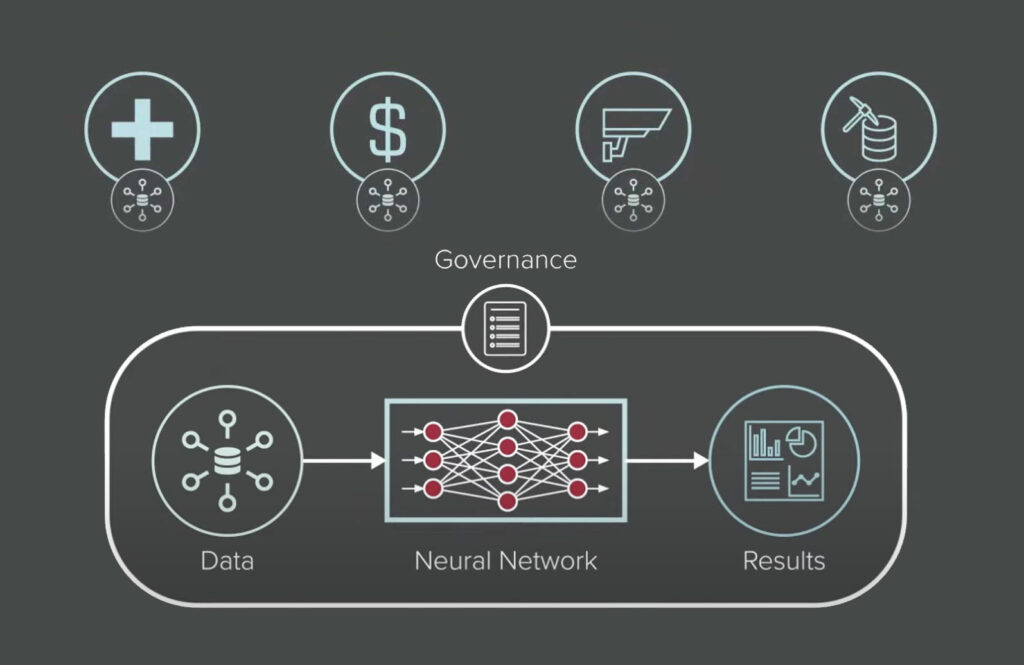
“A.I. co-pilots, assistants and agents promise to boost productivity with helpful suggestions and shortcuts. “
New York Times, September 2024
While this technology is still in the early stages, it’s being hyped as the next big thing in AI, promising to boost productivity and innovation. However, we’re not there yet—agentic AI is mostly a vision for the future that’s rapidly approaching.
Governance Challenges: Accountability, Regulation, and Security
Governance issues with Agentic AI and decentralized computing stem from a lack of centralized control, making regulation and enforcement difficult across jurisdictions. In decentralized systems, no single authority oversees operations, while in Agentic AI, autonomous decisions raise questions about accountability, such as who is responsible when things go wrong—developers, users, or the AI itself.
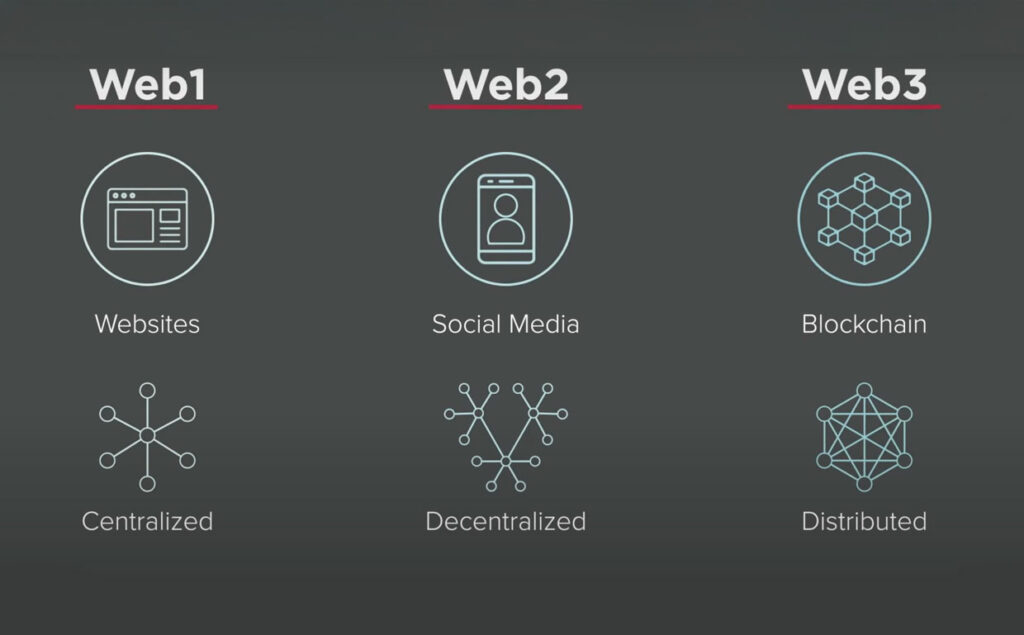
Ethical and legal compliance is challenging since both Agentic AI and decentralized systems may operate beyond traditional frameworks, making it hard to ensure they follow laws or ethical guidelines.
Security is another concern, as decentralized systems may have vulnerabilities from inconsistent protocols, and Agentic AI could be manipulated or develop harmful behaviors. Existing regulatory frameworks are often outdated, creating gaps in oversight for these technologies. Additionally, both technologies struggle with coordination and standardization, as decentralized systems require consensus among many participants, and Agentic AI lacks common standards.
Finally, the lack of transparency in AI decision-making and the difficulty in auditing decentralized systems further complicate governance efforts.
Federated Machine Learning (FedML)
FedML is an approach that allows small-data organizations to train and use sophisticated machine learning models. The definition of small data depends on the complexity of the problem being addressed by AI. In pharma, for example, having access to a million annotated molecules for drug discovery is relatively small in view of the vast chemical space.
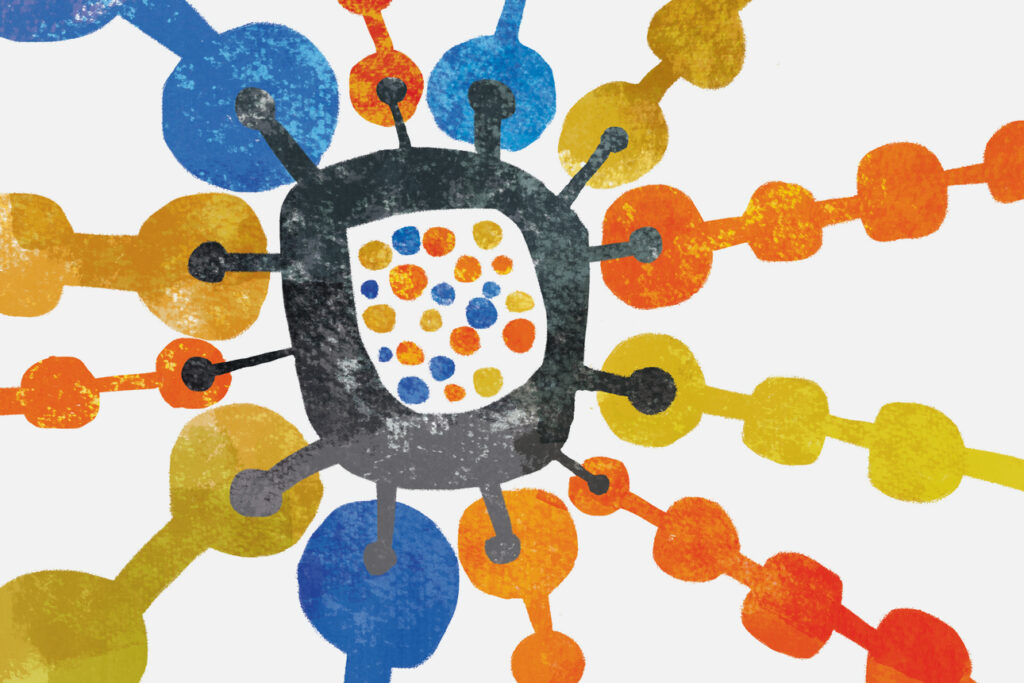
Is Federated Agentic AI the answer?
Federated Agentic AI refers to a blend of two advanced AI concepts: federated learning and agentic AI. Federated learning enables AI models to be trained across decentralized devices or data sources while keeping the data local, enhancing privacy and scalability. Meanwhile, agentic AI involves AI systems that act autonomously, capable of decision-making, planning, and adapting based on real-time data without human intervention.
When combined, Federated Agentic AI allows multiple autonomous agents to collaborate across a distributed network. These agents can handle tasks independently while continuously learning from local data sources, without needing to share sensitive information across the network. This setup is particularly useful in environments like healthcare, finance, or IoT, where data privacy is critical but complex tasks still require intelligent automation.
For instance, a federated agentic system might be deployed in a network of smart devices where each device autonomously manages specific tasks (e.g., optimizing energy use) while learning from local data. These devices can also share insights without revealing user data, improving overall system efficiency and privacy.
Final Thoughts: Designing for new technology is a completely different challenge from a traditional design project.
Typically, users already know how to interact with familiar products—like swiping a credit card at a payment terminal or using a TV remote to change channels. But with emerging technology, there are no familiar cues, making it harder for users to figure out how to engage with it effectively. Think back to when users first encountered smartphones—there was no clear precedent for touchscreens or gestures, making it challenging to learn entirely new interactions.
Adoption of new tech often lags behind confusion and speculation, so creating a seamless, intuitive user experience is essential for success.
That said, designing something brand new isn’t easy, but it’s where we excel as a team. We navigate the space between excitement for new technology and delivering real, user-centered value. By following key principles, we can turn this unfamiliar tech into a product people will love.
For CMOs, this means a potential shift in how to approach marketing and customer engagement. As AI becomes more autonomous, the question will be: how do we control and guide these powerful tools to enhance our strategies while still ensuring privacy? Ultimately it is about using AI in smarter, more effective ways to drive business growth.
Sources:
Analytics Vidhya, The GitHub Blog